引用本文: | 郇嘉嘉,李代猛,杜云飞,沈欣炜,张璇,乔百豪,何春庚,蓝晓东,罗澍忻.基于Prophet算法和Blending集成学习的实时负荷中期预测[J].电力自动化设备,2024,44(4):178-183 |
| HUAN Jiajia,LI Daimeng,DU Yunfei,SHEN Xinwei,ZHANG Xuan,QIAO Baihao,HE Chungeng,LAN Xiaodong,LUO Shuxin.Mid-term forecasting of real-time load based on Prophet algorithm and Blending integrated learning[J].Electric Power Automation Equipment,2024,44(4):178-183 |
|
本文已被:浏览 2002次 下载 375次 |
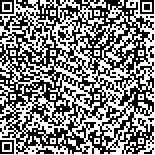 码上扫一扫! |
|
基于Prophet算法和Blending集成学习的实时负荷中期预测 |
郇嘉嘉1, 李代猛2, 杜云飞2, 沈欣炜2, 张璇2, 乔百豪3, 何春庚1, 蓝晓东1, 罗澍忻1
|
1.广东电网有限责任公司电网规划研究中心,广东 广州 510220;2.清华大学深圳国际研究生院,广东 深圳 518055;3.中原工学院 电子信息学院,河南 郑州 451191
|
|
摘要: |
目前的中期负荷预测一般未考虑负荷实时状态,而负荷数据的非线性、季节性、随机性、时序性特征将影响实时负荷的中期预测。构建一个实时负荷中期预测的框架,采用Prophet算法提取负荷数据的季节性部分,采用Blending集成学习对负荷数据的非季节部分进行滚动预测,将季节性部分和非季节性部分合成中期负荷实时数据。爱尔兰电力系统的算例结果验证了模型的有效性和稳定性。 |
关键词: 负荷预测 Prophet算法 Blending集成学习 季节性 |
DOI:10.16081/j.epae.202308025 |
分类号:TM714 |
基金项目:中国南方电网重点科技项目(支撑多能互补园区规划的能效数据挖掘技术研究项目)(GDKJXM20202019) |
|
Mid-term forecasting of real-time load based on Prophet algorithm and Blending integrated learning |
HUAN Jiajia1, LI Daimeng2, DU Yunfei2, SHEN Xinwei2, ZHANG Xuan2, QIAO Baihao3, HE Chungeng1, LAN Xiaodong1, LUO Shuxin1
|
1.Power Grid Planning Research Center of Guangdong Power Grid Co.,Ltd.,Guangzhou 510220, China;2.Tsinghua Shenzhen International Graduate School, Shenzhen 518055, China;3.School of Electronic and Information, Zhongyuan University of Technology, Zhengzhou 451191, China
|
Abstract: |
The current medium-term load forecasting generally doesn’t consider the real-time state of the load. However, the characteristics of load data such as nonlinearity, seasonality, randomness and temporality will influence the medium-term forecasting of real-time load. A framework for mid-term forecasting of real-time load is constructed. The Prophet algorithm is adopted to extract the seasonal component of the load data. The Blending integrated learning is adopted for the rolling forecasting of non-seasonal component of the load data. The seasonal and non-seasonal components are combined to synthesize the real-time data of mid-term load. The effectiveness and stability of the model are verified by Irish Power System. |
Key words: load forecasting Prophet algorithm Blending integrated learning seasonality |